[ad_1]
Summary: Study reveals the brain may not be as efficient as previously believed.
Source: Purdue University
A Purdue University neuroscientist’s findings suggest that the brain is not as efficient as we think.
To be efficient, it’s best to tailor efforts to the final goal. If devices or network connections can’t display or relay high-resolution images, there’s no point in streaming high-definition movies. A less fancy version not only will do, but it will stream faster without blocky artifacts.
In neuroscience, an indicator of the efficiency of relaying information is called sparse coding. Sparse coding means the brain relays (encodes) essential information with just a few (sparse) neurons.
“Entire fields have been based on the assumption that the brain must be perfectly efficient, like a machine, to do its job well,” said Anne Sereno, a professor of psychological sciences and biomedical engineering. “But our research found that this is not always the case.”
This surprising discovery by Sereno and her colleagues is reported in a recent Nature Communications Biology article, “Pseudosparse Neural Coding in the Visual System of Primates.”
Understanding and measuring sparseness
To measure sparseness, Sereno and her team evaluated the data being carried on each neuron. When similar data shows up across many neurons, the team can tell the process is not sparse — it is not efficient. To achieve a high degree of sparseness, each neuron or data-carrying unit must carry or relay a separate piece of information. Neurons need to relay more information with less, without an overlapping of data across various carriers.
A measure called correlation quantifies such overlap. If too many neurons carry similar information, the correlation is high. In the brain, when mechanisms are authentically sparse, there’s no correlation between the neurons.
“Our research examining many different cortical brain regions revealed some interesting insights: When it appears that the brain is conducting sparse coding (using widely accepted measures of sparseness), there is actually significant correlation,” Sereno said.
In such instances, the brain is pseudosparse, or not authentically sparse.
These findings upturn the commonly held belief that the brain is the most efficient mechanism to emulate when designing a variety of engineering applications. At the same time, given that these pseudosparse mechanisms have withstood the evolutionary process over time, perhaps there’s something to be said for sparseness not always being the best way to deliver information. Studying these pseudosparse mechanisms in detail will help scientists understand various brain processes and their relevance to disease and rehabilitation.
Designing engineering processes
So, if the brain is not always efficient, how should scientists approach the design of machines? The answer: Evaluate the task and then pick and choose what to emulate.
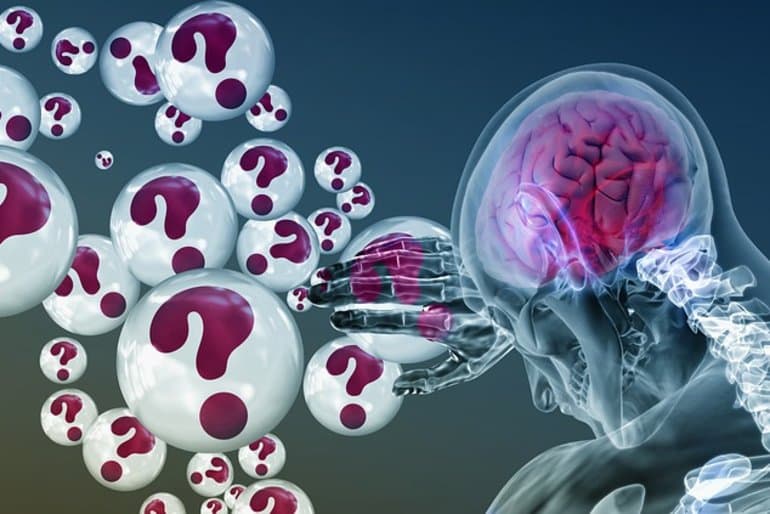
“It is true that in instances where there is a lot of variability or background noise, the brain is better able to concentrate on the task at hand,” Sereno said. “But in instances where there is very little signal interference, using the brain as a model might lead to overdesign.”
As an example, a robotic arm that simply picks and places objects in a controlled environment does not need to worry about noise or signal interference. Its programs can afford to be straightforward and efficient. On the other hand, autonomous driving happens in much more complex environments, with a lot more variability and noise — environments in which the brain excels. In such instances, leaning on the brain for inspiration benefits the eventual design process.
Studying pseudosparseness of brain coding delivers valuable insights not just into how the brain functions but into biomimicry and its effective applications in engineering.
About this neuroscience research news
Source: Purdue University
Contact: Poornima Apte – Purdue University
Image: The image is in the public domain
Original Research: Open access.
“Pseudosparse neural coding in the visual system of primates” by Sidney R. Lehky, Keiji Tanaka & Anne B. Sereno. Nature Communications Biology
Abstract
Pseudosparse neural coding in the visual system of primates
When measuring sparseness in neural populations as an indicator of efficient coding, an implicit assumption is that each stimulus activates a different random set of neurons. In other words, population responses to different stimuli are, on average, uncorrelated.
Here we examine neurophysiological data from four lobes of macaque monkey cortex, including V1, V2, MT, anterior inferotemporal cortex, lateral intraparietal cortex, the frontal eye fields, and perirhinal cortex, to determine how correlated population responses are.
We call the mean correlation the pseudosparseness index, because high pseudosparseness can mimic statistical properties of sparseness without being authentically sparse. In every data set we find high levels of pseudosparseness ranging from 0.59–0.98, substantially greater than the value of 0.00 for authentic sparseness. This was true for synthetic and natural stimuli, as well as for single-electrode and multielectrode data. A model indicates that a key variable producing high pseudosparseness is the standard deviation of spontaneous activity across the population.
Consistently high values of pseudosparseness in the data demand reconsideration of the sparse coding literature as well as consideration of the degree to which authentic sparseness provides a useful framework for understanding neural coding in the cortex.
[ad_2]
Source link